What is Data Analysis?
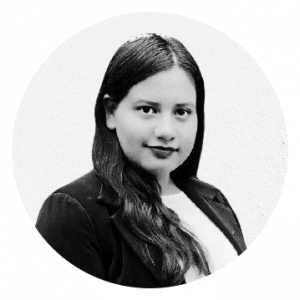
Written by Shrisha Sapkota
Blogger
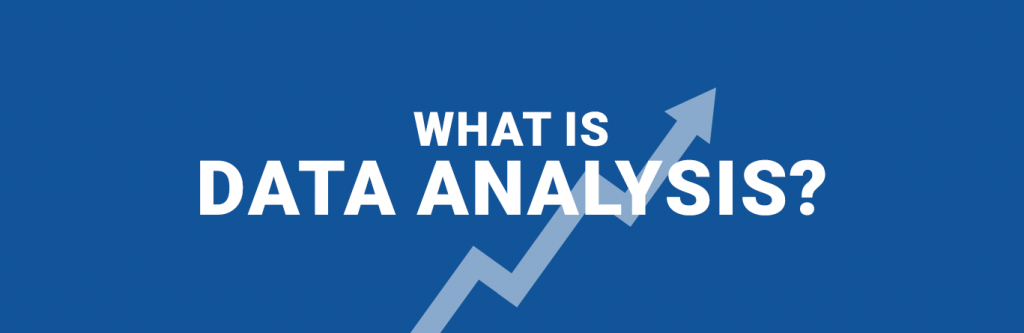
Data has been the buzzword for ages now – either the data being generated from large-scale enterprises or the data generated from an individual, every aspect of data needs to be analysed to benefit yourself from it but how do we do it?[1] This is where the concept of data analysis enters the picture.
Businesses today need every edge and advantage they can get.[2] So, to grow your business – even to grow in your life – sometimes all you need to do is analyse.[3] Thanks to obstacles like rapidly changing markets, economic uncertainty, shifting political landscapes, finicky consumer attitudes, and even global pandemics, businesses right now are working with slimmer margins for error.[4]
If your business is not growing, then you have to look back and acknowledge your mistakes and make a plan again without repeating those mistakes and even if your business is growing, then you have to look forward to making the business grow more – all you need to do is analyse your business data and business processes.[5] In today’s business world, data analysis plays a role in making decisions more scientifically and helping businesses operate more effectively.[6]
Most companies are collecting loads of data all the time—but, in its raw form, this data doesn’t mean anything.[7] It has to be analysed to utilise it and when data is used effectively, it leads to a better understanding of a business’s previous performance and better decision-making for its future activities.[8]
Data analysis is defined as a process of cleaning, transforming, and modelling data to discover useful information for business decision-making.[9] Data analysis has multiple facets and approaches, encompassing diverse techniques under a variety of names, and is used in different business, science, and social science domains.[10]
In our data-rich age, understanding how to analyse and extract true meaning from our business’s digital insights is one of the primary drivers of success.[11] Data Analytics has a key role in improving your business as it is used to gather hidden insights, generate reports, perform market analysis, and improve business requirements.[12]
You can think of data analytics as a form of business intelligence, used to solve specific problems and challenges within an organisation.[13]
In short, data analysis involves sorting through massive amounts of unstructured information and deriving key insights from it and these insights are enormously valuable for decision-making at companies of all sizes.[14]
Here is a simple example of how we use data analysis in our everyday life that will help you understand its concept better: Whenever we make a decision, we always remember the last time we experienced something similar or read, thought, or heard about the topic. We base our decisions on these factors as they give us a picture of what might happen in a situation where we pick that option. This is similar to data analysis. An analyst does the same thing for business purposes, calling it Data Analysis.[15]
No one makes important decisions without first finding out what’s at stake, the pros, and cons, and the possible outcomes – similarly, no company that wants to succeed should make decisions based on bad data – organisations need information; they need data.[16]
In science, data analysis uses a more complex approach with advanced techniques to explore and experiment with data.[17] While data analysis in qualitative research can include statistical procedures, many times analysis becomes an ongoing iterative process where data is continuously collected and analysed almost simultaneously.[18]
Despite the colossal volume of data we create every day, a mere 0.5% is analysed and used for data discovery, improvement, and intelligence – while that may not seem like much, considering the amount of digital information we have at our fingertips, half a per cent still accounts for a vast amount of data.[19] Armed with the insights drawn from the data, businesses, and organisations can develop a much deeper understanding of their audience, their industry, and their company as a whole—and, as a result, are much better equipped to make decisions and plan.[20]
Researchers generally analyse for patterns in observations through the entire data collection phase[21] – finding patterns in a dataset that can tell you something useful and relevant about a particular area of the business—how certain customer groups behave, for example, or how employees engage with a particular tool.[22]
Data Analysis Vs Data Science – What is the Difference?
The upsurge of Big Data – which includes everything we do online like: texts, emails, tweets, user searches (on search engines), social media chatter, data generated from IoT, and connected devices – has brought along two buzzwords in the industry, Data Science and Data Analytics.[23] Dan Ariely, a well-known behavioural economics expert, once said about big data: “Everyone talks about it, nobody knows how to do it, everyone thinks everyone else is doing it, so everyone claims they are doing it.”[24]
You’ll find that the terms “data science” and “data analytics” tend to be used interchangeably but they are two different fields and denote two distinct career paths that each have a very different impact on the business or organisation.[25] The confusion primarily arises from the fact that both Data Scientists and Data Analysts work with Big Data – even so, the difference between Data Science vs. Data Analytics is stark.[26]
Data science is a concept used to tackle big data and includes data cleansing, preparation, and analysis.[27] Data science focuses on discovering new questions that you might not have realised needed answering to drive innovation.[28] It includes concepts like data mining, data inference, predictive modelling, and ML algorithm development, to extract patterns from complex datasets and transform them into actionable business strategies.[29] A data scientist gathers data from multiple sources and applies machine learning, predictive analytics, and sentiment analysis to extract critical information from the collected data sets – essentially, they understand data from a business point of view and can provide accurate predictions and insights that can be used to power critical business decisions.[30]
Data analytics focuses more on viewing the historical data in context while data science focuses more on machine learning and predictive modelling.[31] It is designed to uncover the specifics of extracted insights.[32]
It involves answering questions generated for better business decision-making.[33] They examine large datasets intending to identify trends and patterns, then “visualise” their findings in the form of charts, graphs, and dashboards which are shared with key stakeholders and used to make informed data-driven strategic decisions.[34]
Types of Data Analysis
The kinds of insights you get from your data depend on the type of analysis you perform.[35] Data analytics is a broad field.[36] Every kind of data has a rare quality of describing things after assigning a specific value to it – so for analysis, you need to organise these values, processed and presented in a given context, to make it useful.[37]
The four major types of analysis are: descriptive, diagnostic, predictive, and prescriptive.[38] Each type has a different goal and a different place in the data analysis process.[39]
Descriptive analytics
Descriptive analytics is a simple, surface-level type of analysis that looks at what has happened in the past.[40] It is designed to answer the question “What happened?”[41] These techniques summarise large datasets to describe outcomes to stakeholders.[42] It is at the foundation of all data insight and is the simplest and most common use of data in business today.[43] Examples include monthly revenue reports and KPI dashboards.[44]
Diagnostic analytics
While descriptive analytics looks at the “what”, diagnostic analytics explores the “why”.[45] It takes the insights found from both descriptive and exploratory analytics and investigates further to find the causes.[46] These techniques supplement more basic descriptive analytics and take the findings from descriptive analytics and dig deeper to find the cause.[47] Organisations make use of this type of analytics as it creates more connections between data and identifies patterns of behaviour.[48]
Predictive analytics
Just as the name suggests, predictive analytics tries to predict what is likely to happen in the future.[49] It uses data, statistics, and machine learning algorithms and techniques to figure out the likelihood of future outcomes based on data and is often used more by data scientists, rather than data analysts.[50] This type of analysis is another step up from the descriptive and diagnostic analysis as it relies on statistical modelling, which requires added technology and manpower to forecast.[51] The accuracy of the predictions depends on the input variables and the types of models – a linear model might work well in some cases, and vice-versa.[52] Examples include sales forecasting and risk assessment.[53]
Prescriptive analytics
Building on predictive analytics, prescriptive analytics advises on the actions and decisions that should be taken.[54] It helps answer questions about what should be done and by using its insights, data-driven decisions can be made which allows businesses to make informed decisions in the face of uncertainty.[55] It is the frontier of data analysis[56] as it takes insights found from all types of data analysis (descriptive, exploratory, diagnostic, predictive) to determine the best course of action.[57]
Law and Data Analytics – Legal Analytics
Big data analytics technologies are becoming omnipresent in many industries, and the legal sector is no exception.[58] The legal industry is still in a very early stage of the adoption of legal data analytics; however, most law firms or corporate legal departments now acknowledge the importance of better data capture and recognize that their focus in this area should extend beyond mere financial data.[59] The legal practices that embrace data-driven thinking now are putting themselves in a position to excel relative to their competitors that aren’t.[60]
Data analytics for law firms is the application of data to the business and practice of law that harnesses technologies, such as machine learning, artificial intelligence, and searching, to clean up, structure, and analyse raw data from dockets and other legal documents.[61] In practice, data analytics for lawyers tools are helping them make data-driven decisions on which to build their legal strategies which could mean things like knowing the probability of a specific motion outcome, how seemingly unrelated cases connect or how much a settlement award could be.[62] The benefits of law firms’ data analytics include saving time, reducing risk, improving decision-making, and increasing goodwill and trust from clients.[63]
A proper appreciation for and collection of data, such as capturing outcome and settlement data for all litigation matters, helps position an organisation for artificial intelligence and advanced analytics, and as advanced electronic document storage in the legal sector grows, so will the latent value within that collected data.[64] Recently, we have also seen the use of data analytics in the legal industry in judicial opinions to predict how specific judges may decide cases, including providing recommendations on specific precedents and language that may appeal to a given judge.[65]
To a lawyer, legal practice analytics software can offer some pretty powerful insight which could be something like the probability of a motion outcome or a rough dollar amount of a verdict or settlement but the value of legal industry analytics goes way, way deeper than that.[66] There are many functions outside the practice of law in any law firm, including marketing as at its core, a law firm is a business providing a service and successful businesses use data to understand and target their customer base more effectively; expand communications into expert industries; monitor the effectiveness of marketing campaigns; build a better understanding of business needs, and identify areas that are worth continuous investing in. [67]
By assessing some data points – outcomes from previous cases; the number of judgments or settlements; the overall costs of similar matters – data analysis can give a lawyer or a legal team an objective answer to this question based on facts and historical data.[68] Legal service analytics can also help you better compete with other law firms, from coming up with a winning pitch to selling your expertise in specific practice areas, it allows you to compile hard data about your success that you can use to show prospective clients exactly why you are the best in the business.[69]
While some might still perceive legal analytics software as a threat to the traditional legal sector, it also presents numerous opportunities to capitalise on emerging trends and allow lawyers to focus on the intellectually engaging aspects of law, instead of the mundane and tedious and there is no doubt this will ultimately improve the profession of law, for both lawyers and our clients.[70] Data analytics is already helping lawyers make smarter, data-driven decisions—and helping them do it faster which tells us that the proliferation of related analytics technologies will only accelerate in the future.[71]
References
[1]https://www.edureka.co/blog/what-is-data-analytics/#:~:text=Data%20Analytics%20refers%20to%20the,to%20the%20organization%20or%20individual.
[2] https://www.simplilearn.com/data-analysis-methods-process-types-article
[3] https://www.guru99.com/what-is-data-analysis.html
[4] https://www.simplilearn.com/data-analysis-methods-process-types-article
[5] https://www.guru99.com/what-is-data-analysis.html
[6] https://en.wikipedia.org/wiki/Data_analysis
[7] https://careerfoundry.com/en/blog/data-analytics/what-is-data-analytics/
[8] https://chartio.com/learn/data-analytics/types-of-data-analysis/
[9] https://www.guru99.com/what-is-data-analysis.html
[10] https://en.wikipedia.org/wiki/Data_analysis
[11] https://www.datapine.com/blog/data-analysis-methods-and-techniques/
[12]https://www.edureka.co/blog/what-is-data-analytics/#:~:text=Data%20Analytics%20refers%20to%20the,to%20the%20organization%20or%20individual.
[13] https://careerfoundry.com/en/blog/data-analytics/what-is-data-analytics/
[14] https://learntocodewith.me/posts/data-analysis/
[15] https://www.guru99.com/what-is-data-analysis.html
[16] https://www.simplilearn.com/data-analysis-methods-process-types-article
[17] https://www.datapine.com/blog/data-analysis-methods-and-techniques/
[18] https://ori.hhs.gov/education/products/n_illinois_u/datamanagement/datopic.html
[19] https://www.datapine.com/blog/data-analysis-methods-and-techniques/
[20] https://careerfoundry.com/en/blog/data-analytics/what-is-data-analytics/
[21] https://ori.hhs.gov/education/products/n_illinois_u/datamanagement/datopic.html
[22] https://careerfoundry.com/en/blog/data-analytics/what-is-data-analytics/
[23] https://www.upgrad.com/blog/data-science-vs-data-analytics/
[24] https://www.northeastern.edu/graduate/blog/data-analytics-vs-data-science/
[25] https://careerfoundry.com/en/blog/data-analytics/what-is-data-analytics/
[26] https://www.upgrad.com/blog/data-science-vs-data-analytics/
[27]https://www.simplilearn.com/data-science-vs-data-analytics-vs-machine-learning-article#:~:text=While%20a%20data%20scientist%20is,the%20existing%20set%20of%20questions.
[28]https://medium.com/@springboard_ind/data-science-vs-data-analytics-how-to-decide-which-one-is-right-for-you-41e7bdec080e
[29] https://www.upgrad.com/blog/data-science-vs-data-analytics/
[30]https://www.simplilearn.com/data-science-vs-data-analytics-vs-machine-learning-article#:~:text=While%20a%20data%20scientist%20is,the%20existing%20set%20of%20questions.
[31]https://medium.com/@springboard_ind/data-science-vs-data-analytics-how-to-decide-which-one-is-right-for-you-41e7bdec080e
[32] https://www.upgrad.com/blog/data-science-vs-data-analytics/
[33]https://medium.com/@springboard_ind/data-science-vs-data-analytics-how-to-decide-which-one-is-right-for-you-41e7bdec080e
[34] https://www.upgrad.com/blog/data-science-vs-data-analytics/
[35] https://careerfoundry.com/en/blog/data-analytics/different-types-of-data-analysis/
[36] https://www.mastersindatascience.org/learning/what-is-data-analytics/
[37] https://www.questionpro.com/blog/data-analysis-in-research/
[38] https://careerfoundry.com/en/blog/data-analytics/different-types-of-data-analysis/
[39] https://www.mastersindatascience.org/learning/what-is-data-analytics/
[40] https://careerfoundry.com/en/blog/data-analytics/what-is-data-analytics/
[41] https://learntocodewith.me/posts/data-analysis/
[42] https://www.mastersindatascience.org/learning/what-is-data-analytics/
[43] https://chartio.com/learn/data-analytics/types-of-data-analysis/
[44] https://learntocodewith.me/posts/data-analysis/
[45] https://careerfoundry.com/en/blog/data-analytics/what-is-data-analytics/
[46] https://learntocodewith.me/posts/data-analysis/
[47] https://www.mastersindatascience.org/learning/what-is-data-analytics/
[48] https://chartio.com/learn/data-analytics/types-of-data-analysis/
[49] https://careerfoundry.com/en/blog/data-analytics/what-is-data-analytics/
[50] https://learntocodewith.me/posts/data-analysis/
[51] https://chartio.com/learn/data-analytics/types-of-data-analysis/#diagnostic-analysis
[52] https://towardsdatascience.com/the-six-types-of-data-analysis-75517ba7ea61
[53] https://learntocodewith.me/posts/data-analysis/
[54] https://careerfoundry.com/en/blog/data-analytics/what-is-data-analytics/
[55] https://www.mastersindatascience.org/learning/what-is-data-analytics/
[56] https://chartio.com/learn/data-analytics/types-of-data-analysis/#diagnostic-analysis
[57] https://learntocodewith.me/posts/data-analysis/
[58]https://www.lexisnexis.ca/sites/en-ca/sl/2020-03/what-is-legal-analytics.page#:~:text=Put%20simply%2C%20legal%20analytics%20is,to%20build%20their%20legal
[59] https://www.landers.com.au/legal-insights-news/the-future-of-analytics-in-legal
[60]https://legal.thomsonreuters.com/en/insights/articles/five-common-legal-questions-that-predictive-data-analytics-can-help-answer
[61] https://guides.libraries.uc.edu/legalanalytics
[62]https://www.lexisnexis.ca/sites/en-ca/sl/2020-03/what-is-legal-analytics.page#:~:text=Put%20simply%2C%20legal%20analytics%20is,to%20build%20their%20legal
[63] https://lawrina.com/blog/how-attorneys-use-legal-analytics/
[64] https://www.landers.com.au/legal-insights-news/the-future-of-analytics-in-legal
[65]https://seleritysas.com/blog/2020/10/02/how-ai-and-big-data-analytics-will-revolutionise-the-legal-system/
[66]https://www.lexisnexis.ca/sites/en-ca/sl/2020-03/what-is-legal-analytics.page#:~:text=Put%20simply%2C%20legal%20analytics%20is,to%20build%20their%20legal
[67] https://www.promediate.co.uk/how-data-analytics-is-transforming-the-legal-industry/
[68]https://legal.thomsonreuters.com/en/insights/articles/five-common-legal-questions-that-predictive-data-analytics-can-help-answer
[69] https://www.promediate.co.uk/how-data-analytics-is-transforming-the-legal-industry/
[70] https://www.landers.com.au/legal-insights-news/the-future-of-analytics-in-legal
[71]https://www.lexisnexis.ca/sites/en-ca/sl/2020-03/what-is-legal-analytics.page#:~:text=Put%20simply%2C%20legal%20analytics%20is,to%20build%20their%20legal